Transforming Agriculture with Machine Learning: Harnessing the Power of Agricultural Datasets
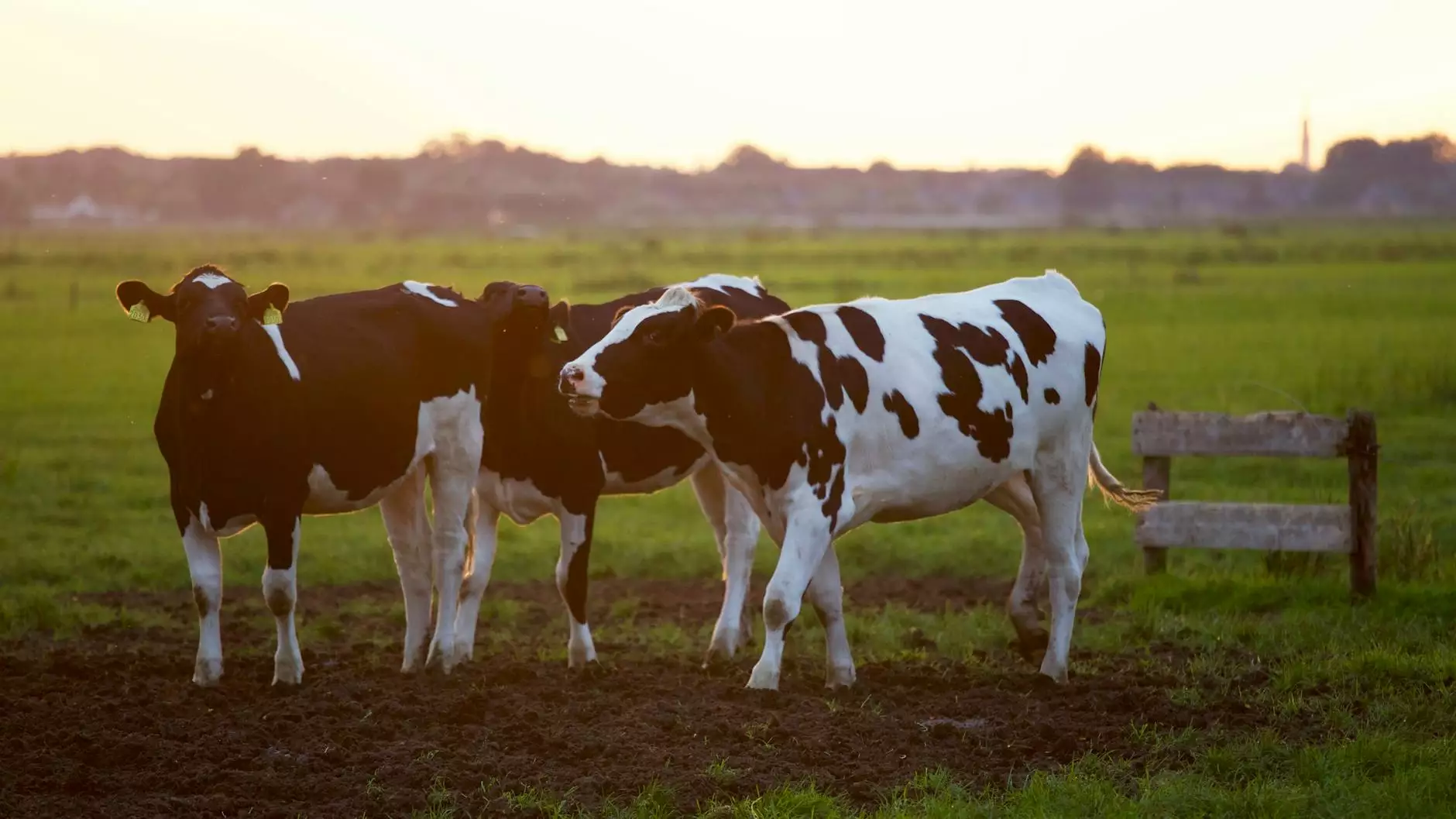
(1) Understanding the Importance of Agricultural Datasets
In the modern agricultural landscape, data plays a pivotal role in decision-making and sustainability. The rich insights derived from an agriculture dataset for machine learning are invaluable to farmers and agribusinesses alike. These datasets encompass a variety of information, from weather patterns and soil conditions to crop yields and pest infestations, providing a comprehensive view that enables better planning and execution.
(2) Machine Learning: A Game Changer for Agriculture
The advent of machine learning technologies has opened up new avenues for optimizing agricultural practices. By leveraging an agriculture dataset for machine learning, stakeholders can develop predictive models that enhance yield forecasts, minimize resource waste, and improve the overall efficiency of farming operations.
(2.1) Predictive Analytics in Crop Management
Predictive analytics is one of the applications of machine learning that significantly impacts crop management. Utilizing an agriculture dataset for machine learning, farmers can analyze historical data to predict future outcomes based on various influencing factors. For instance, they can forecast the best times for planting and harvesting, thus maximizing yield and minimizing losses.
(3) Key Components of Agriculture Datasets
Understanding the components of agricultural datasets is crucial for effective utilization in machine learning. These datasets typically include:
- Soil Properties: Nutrient content, pH levels, and moisture levels.
- Weather Data: Temperature, rainfall patterns, and humidity.
- Crop Varieties: Different species and their performance metrics.
- Pest and Disease Information: Historical data on infestations and outbreaks.
- Yield Records: Past harvests and productivity levels.
(4) The Role of Data Collection Technologies
Advanced data collection technologies such as IoT devices, drones, and satellite imagery have revolutionized the agricultural sector. These technologies facilitate the gathering of extensive datasets that can feed into machine learning algorithms, thus enhancing the quality and accuracy of insights derived from the data.
(4.1) IoT Devices and their Impact
The Internet of Things (IoT) provides a network of connected devices that measure various environmental parameters. This real-time data collection feeds directly into an agriculture dataset for machine learning, allowing farmers to monitor crop health and soil conditions dynamically.
(4.2) Drones and Satellite Imagery
Drones equipped with high-resolution cameras and sensors can capture detailed images and data of agricultural fields. When integrated with machine learning platforms, these datasets can help farmers visualize crop health, monitor irrigation efficiency, and identify areas needing attention.
(5) Enhancing Decision Making Through Data Visualization
Visualization tools are crucial for interpreting the results of machine learning analyses. By presenting data in an easily understandable format, stakeholders can make more informed decisions. Effective data visualization transforms complex analytics derived from an agriculture dataset for machine learning into actionable insights.
(5.1) Dashboard Technologies
Dashboard technologies aggregate data from multiple sources, offering real-time updates and visualizations. This allows farmers to quickly grasp the health of their crops, monitor trends, and respond proactively to any issues.
(6) Challenges and Considerations in Utilizing Agricultural Datasets
While leveraging an agriculture dataset for machine learning brings numerous benefits, it also presents challenges. These challenges include:
- Data Quality: Ensuring that collected data is accurate and relevant is paramount.
- Data Integration: Merging datasets from various sources can be complex.
- Privacy Concerns: Addressing data ownership and privacy issues is essential.
- Cost of Technology: The initial investment for advanced technologies can be a barrier for some farmers.
(7) Future of Agriculture with Machine Learning
The future of agriculture is increasingly intertwined with machine learning. As technology advances and datasets become more robust, the potential applications in farming will only continue to expand. From precision farming to automated decision-making processes, the integration of machine learning will fundamentally reshape the agricultural sector.
(7.1) Sustainable Farming Practices
One of the most exciting prospects is the potential for sustainable practices. By marrying machine learning insights with sustainable agricultural techniques, farmers can optimize resource use, reduce waste, and minimize environmental impact while maintaining productivity. This will help ensure food security for the growing global population.
(8) Conclusion: Embracing the Data-Driven Revolution in Agriculture
The use of an agriculture dataset for machine learning represents a transformational opportunity for the agricultural industry. As stakeholders embrace data-driven practices, they unlock new efficiencies, enhance yields, and contribute to a more sustainable future in farming. The journey toward data-centric agriculture is well underway, and it is essential for all stakeholders to engage with and adapt to this technological evolution.
For more insights on home services, keys, and locksmiths, visit keymakr.com for an array of solutions to your needs.